Interactive Multi-Dimension Modulation for Image Restoration
Jingwen He, Chao Dong, Liu Yihao, Yu Qiao
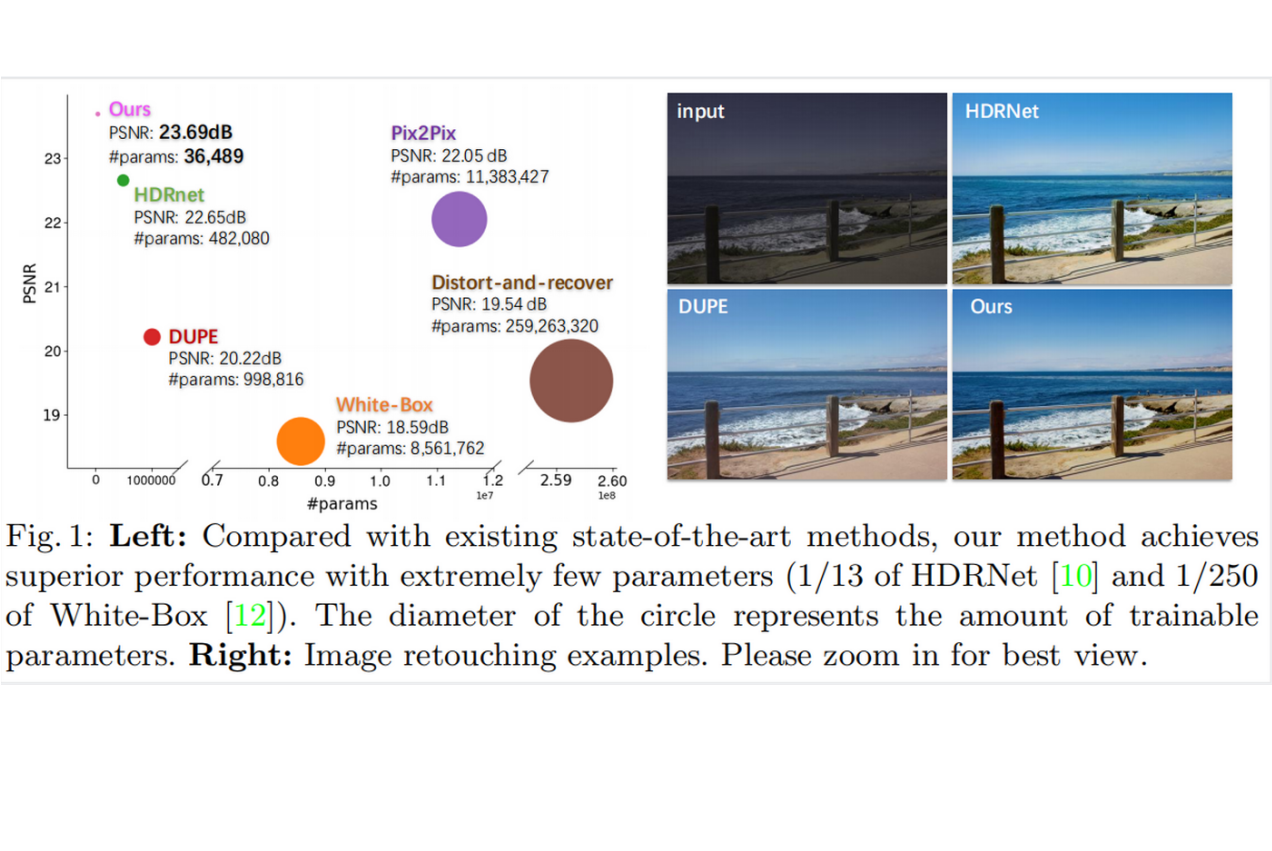
Interactive image restoration aims to generate restored images by adjusting a controlling coefficient which determines the restoration level. Previous works are restricted in modulating image with a single coefficient. However, real images always contain multiple types of degradation, which cannot be well determined by one coefficient. This paper presents a new problem setup, called multi-dimension (MD) modulation, which aims at modulating output effects across multiple degradation types and levels. Compared with the previous single-dimension (SD) modulation, the MD setup to handle multiple degradations adaptively and relief data unbalancing problem in different degradation types. We also propose a deep architecture - CResMD with newly introduced controllable residual connections. Specifically, we add a controlling variable on the conventional residual connection to allow a weighted summation of input and residual. The values of these weights are generated by another condition network. We further propose a new data sampling strategy based on beta distribution together with a simple loss reweighting approach to balance different degradation types and levels. With corrupted image and degradation information as inputs, the network can output the corresponding restored image. By tweaking the condition vector, users can control the output effects in MD space at test time.